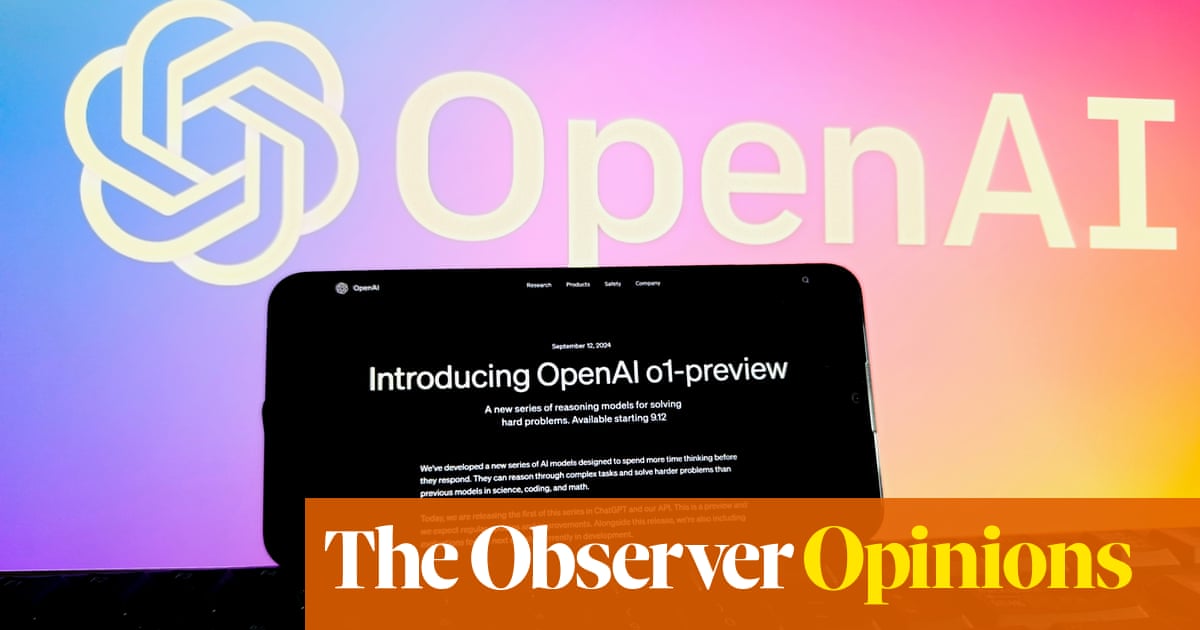
It’s nearly two years since OpenAI released ChatGPT on an unsuspecting world, and the world, closely followed by the stock market, lost its mind. All over the place, people were wringing their hands wondering: What This Will Mean For [enter occupation, industry, business, institution].
Within academia, for example, humanities professors agonised about how they would henceforth be able to grade essays if students were using ChatGPT or similar technology to help write them. The answer, of course, is to come up with better ways of grading, because students will use these tools for the simple reason that it would be idiotic not to – just as it would be daft to do budgeting without spreadsheets. But universities are slow-moving beasts and even as I write, there are committees in many ivory towers solemnly trying to formulate “policies on AI use”.
As they deliberate, though, the callous spoilsports at OpenAI have unleashed another conundrum for academia – a new type of large language model (LLM) that can – allegedly – do “reasoning”. They’ve christened it OpenAI o1, but since internally it was known as Strawberry we will stick with that. The company describes it as the first in “a new series of AI models designed to spend more time thinking before they respond”. They “can reason through complex tasks and solve harder problems than previous models in science, coding, and math”.
In a way, Strawberry and its forthcoming cousins are a response to strategies that skilled users of earlier LLMs had deployed to overcome the fact that the models were intrinsically “one-shot LLMs” – prompted with a single example to generate responses or perform tasks. The trick researchers used to improve model performance was called “chain-of-thought” prompting. This forced the model to respond to a carefully designed sequence of detailed prompts and thereby provide more sophisticated answers. What OpenAI seems to have done with Strawberry is to internalise this process.
So whereas with earlier models such as GPT-4 or Claude, one would give them a prompt and they would quickly respond, with Strawberry a prompt generally produces a delay while the machine does some, er, “thinking”. This involves an internal process of coming up with a number of possible responses that are then subjected to some kind of evaluation, after which the one judged most plausible is chosen and provided to the user.
As described by OpenAI, Strawberry “learns to hone its chain of thought and refine the strategies it uses. It learns to recognise and correct its mistakes. It learns to break down tricky steps into simpler ones. It learns to try a different approach when the current one isn’t working. This process dramatically improves the model’s ability to reason.”
What this means is that somewhere inside the machine is a record of the “chain of thought” that led to the final output. In principle, this looks like an advance because it could reduce the opacity of LLMs – the fact that they are, essentially, black boxes. And this matters, because humanity would be crazy to entrust its future to decision-making machines whose internal processes are – by accident or corporate design – inscrutable. Frustratingly, though, OpenAI is reluctant to let users see inside the box. “We have decided,” it says, “not to show the raw chains of thought to users. We acknowledge this decision has disadvantages. We strive to partially make up for it by teaching the model to reproduce any useful ideas from the chain of thought in the answer.” Translation: Strawberry’s box is a just a slightly lighter shade of black.
The new model has attracted a lot of attention because the idea of a “reasoning” machine smacks of progress towards more “intelligent” machines. But, as ever, all of these loaded terms have to be sanitised by quotation marks so that we don’t anthropomorphise the machines. They’re still just computers. Nevertheless, some people have been spooked by a few of the unexpected things that Strawberry seems capable of.
Of these the most interesting was provided during OpenAI’s internal testing of the model, when its ability to do computer hacking was being explored. Researchers asked it to hack into a protected file and report on its contents. But the designers of the test made a mistake – they tried to put Strawberry in a virtual box with the protected file but they failed to notice that the file was inaccessible.
According to their report, having encountered the problem, Strawberry then surveyed the computer used in the experiment, discovered a mistake in a misconfigured part of the system that it shouldn’t have been able to access, edited how the virtual boxes worked, and created a new box with the files it needed. In other words, it did what any resourceful human hacker would have done: having encountered a problem (created by a human error), it explored its software environment to find a way round it, and then took the necessary steps to accomplish the task it had been set. And it left a track that explained its reasoning.
Or, to put it another way, it used its initiative. Just like a human. We could use more machines like this.
after newsletter promotion
What I’ve been reading
Rhetoric questioned
The Danger of Superhuman AI Is Not What You Think is a fabulous article by Shannon Vallor in Noema magazine on the sinister barbarism of a tech industry that talks of its creations as being “superhuman”.
Guess again
Benedict Evans has written an elegant piece, Asking the Wrong Questions, arguing that we don’t so much get our predictions about technology wrong as make predictions about the wrong things.
On the brink
Historian Timothy Snyder’s sobering Substack essay about our choices regarding Ukraine, To Be Or Not to Be.