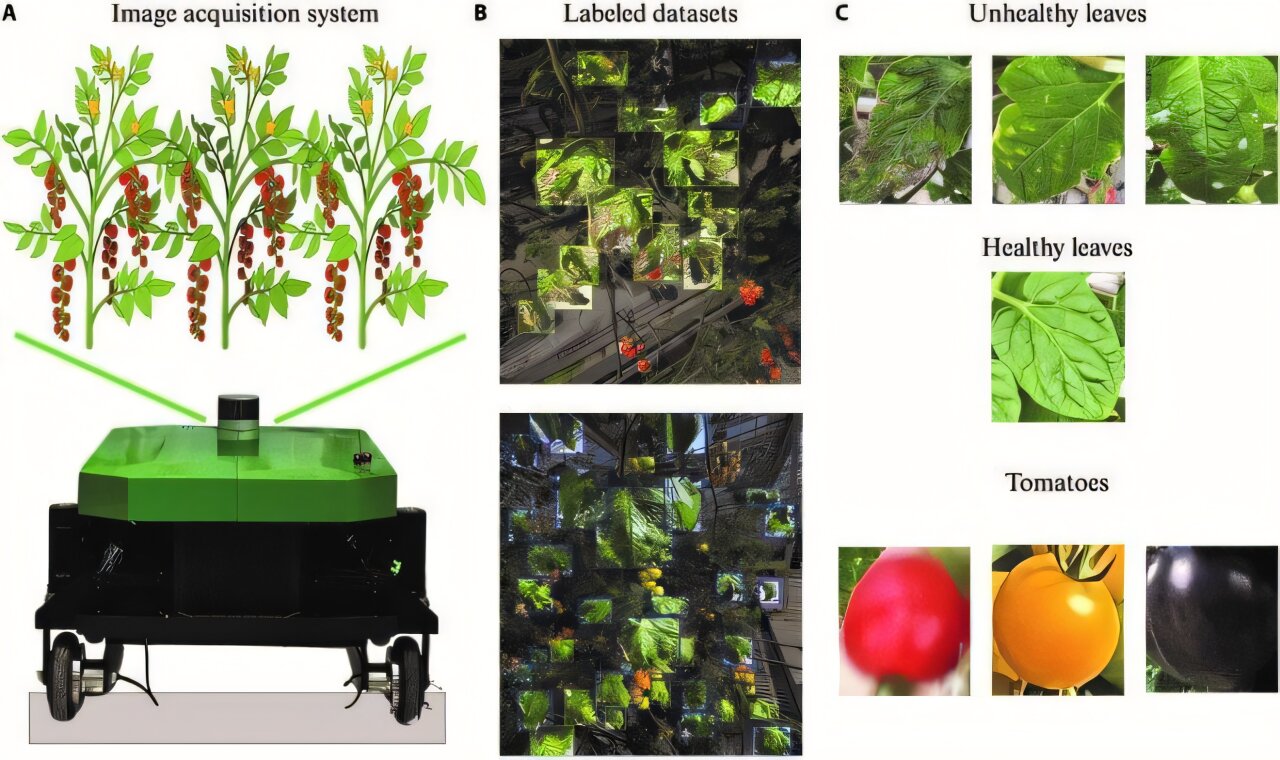
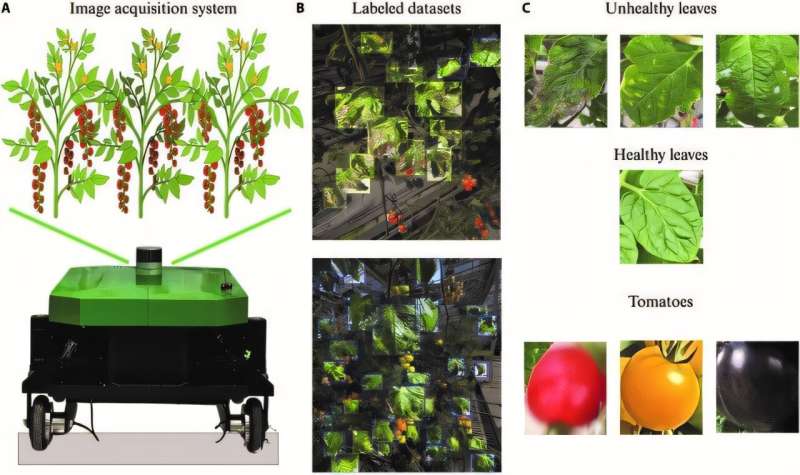
Tomatoes are a critical source of nutrients and remain one of the most widely cultivated fruits globally. However, intensive greenhouse practices increase susceptibility to diseases, which can reduce yields by up to 30% and degrade fruit quality.
Traditional methods of plant monitoring are labor-intensive and often ineffective in real-world conditions due to environmental challenges like varying lighting and background complexity. A study published in Plant Phenomics revolutionizes greenhouse management and prevents disease spread.
By integrating disease detection and fruit counting in real time, this innovation promises to enhance sustainable production practices for one of the world’s most vital crops.
The research utilized YOLOX, NanoDet, and YOLO-TGI algorithms to evaluate the accuracy of tomato disease detection and fruit counting. Various detectors were tested on a comprehensive dataset, and confidence distributions for unhealthy leaves, healthy leaves, and tomatoes were visualized using violin plots.
Results showed that increased network complexity generally improved detection accuracy. Specifically, YOLOX-M achieved optimal performance, with leaf detection confidence scores concentrated around 0.9, while YOLOX-N demonstrated weaker performance, with scores ranging from 0.2 to 0.7, likely due to its lightweight structure.
Both NanoDet and YOLO-TGI displayed similar trends, maintaining robust scores above 0.4. The YOLOX model had the fastest inference speed at 32.35 ms and the highest mean average precision (mAP) of 0.85, while NanoDet featured the smallest model size, and YOLO-TGI achieved the lowest FLOPs and checkpoint weights.
For fruit tracking, the YOLO-TGI-S with Byte-Track combination delivered superior performance, with an R2 of 0.93 and an RMSE of 9.17. In contrast, NanoDet-S and FairMot recorded the lowest R2 of 0.34. Challenges included occlusions, environmental lighting variations, and ID-switching in video streams, mitigated using an ID-filtering strategy.
Overall, YOLO-TGI-S paired with Byte-Track emerged as the most effective configuration, offering a high-speed and accurate solution for real-time monitoring of tomato plant growth and yield estimation.
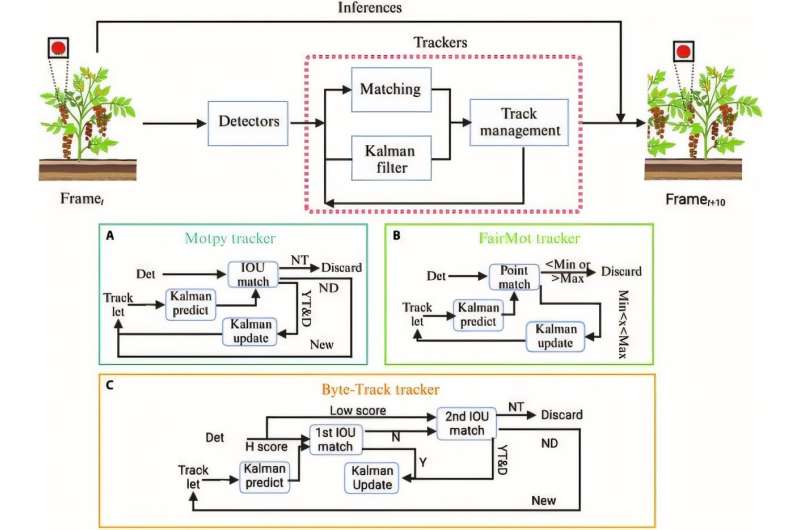
According to the study’s senior researcher, Dr. Shangpeng Sun, “Early detection of diseases and accurate yield estimation are pivotal for sustainable agriculture. Our integrated system represents a significant step forward, offering high-speed and precise monitoring that can be adapted to multiple crops beyond tomatoes.”
This research marks a breakthrough in automated agricultural monitoring. By offering a scalable, efficient, and high-speed solution for disease detection and yield assessment, the system has the potential to transform modern farming practices and support global food security efforts.
More information:
Rui Kang et al, Toward Real Scenery: A Lightweight Tomato Growth Inspection Algorithm for Leaf Disease Detection and Fruit Counting, Plant Phenomics (2024). DOI: 10.34133/plantphenomics.0174
Provided by
Chinese Academy of Sciences
Citation:
Smart surveillance system improves tomato plant monitoring with high-speed disease detection, fruit counting (2024, November 11)
retrieved 11 November 2024
from https://phys.org/news/2024-11-smart-surveillance-tomato-high-disease.html
This document is subject to copyright. Apart from any fair dealing for the purpose of private study or research, no
part may be reproduced without the written permission. The content is provided for information purposes only.