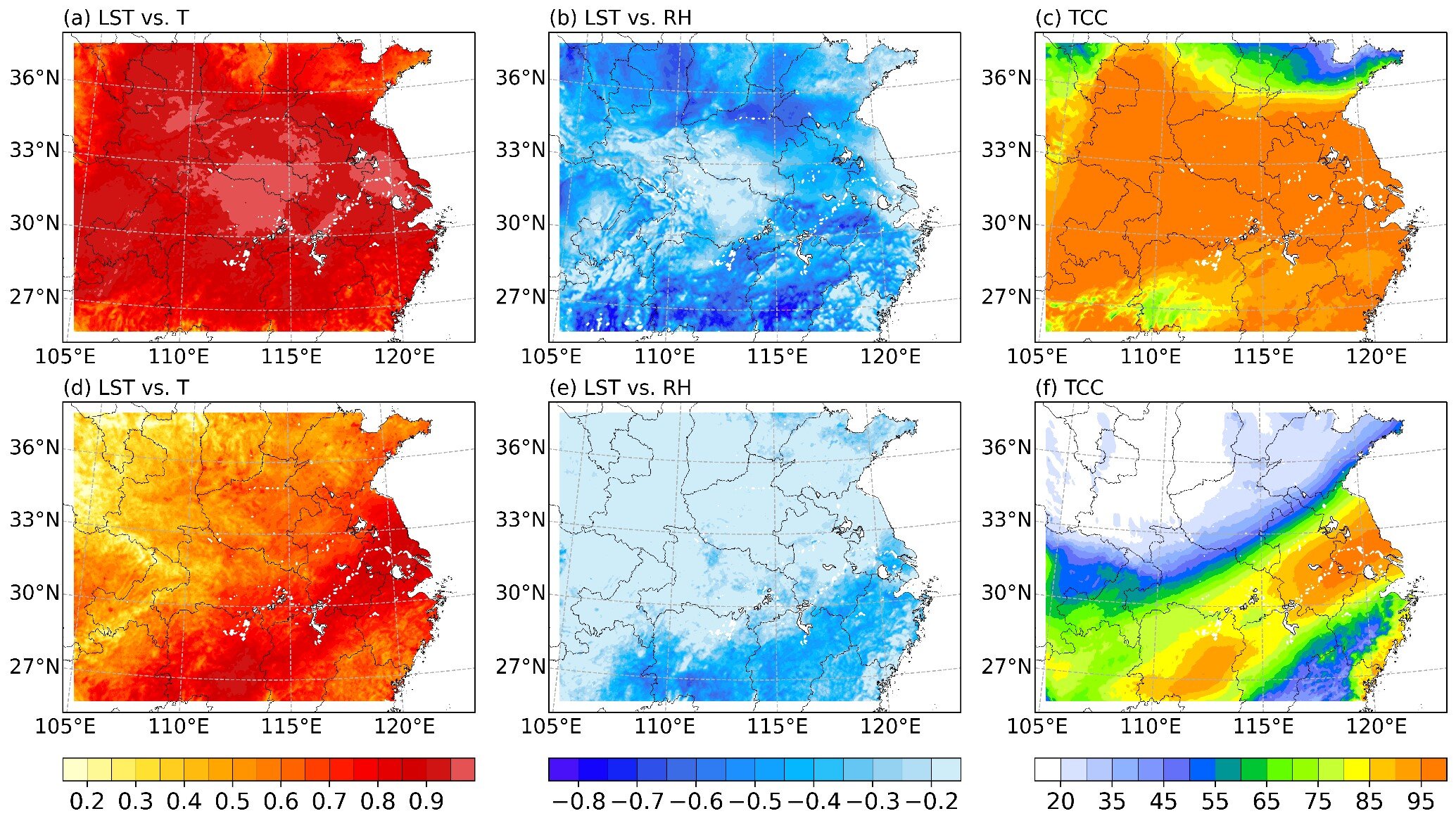
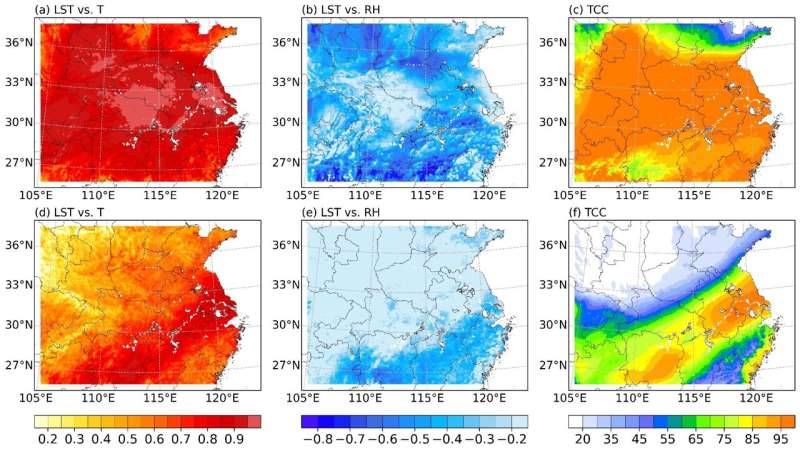
Land surface temperature (LST) is the key variable in land–atmosphere interaction, having an important impact on weather and climate forecasting. Although there have been advances in data assimilation within land-atmosphere coupled models, weakly coupled assimilation remains predominant. This means that the cross-component interactions between land and atmosphere are not adequately considered during the assimilation process, making it difficult to achieve consistent analysis between the land and atmospheric variables.
Professor Yaodeng Chen’s team at Nanjing University of Information Science & Technology (NUIST) has constructed a coupled background error covariance that crosses the land and atmospheric components to address the critical issue of strongly coupled land-atmosphere data assimilation. Their work reveals the connection between the background error of surface and atmospheric variables, and has been published in Atmospheric and Oceanic Science Letters.
Firstly, the study generated the climatological and ensemble forecast samples based on the 2023 mei-yu season, and then the cross-component background error covariance was calculated using forecast error estimation techniques. The results of the study show that the error of LST was mainly correlated with the atmospheric temperature, the correlation decreased with an increase in atmospheric altitude, and the error of the standard deviation of the LST was noticeably larger than that of the low-level atmospheric temperature.
The team also selected two days with large differences in weather conditions for characterization, and the results showed that the error correlation and error standard deviation of the LST and low-level atmospheric temperature and humidity were closely related to the weather background, with obvious diurnal variations.
The research team plans to explore the impact of different seasons on the results in subsequent studies and further apply these findings to strongly coupled land-atmosphere data assimilation. These studies will help to further improve the forecast skill of land-atmosphere coupled models, providing more accurate information support for weather forecasting and early warning.
More information:
Qihang Yang et al, Construction and characteristic analysis of background error covariance coupled with land surface temperature, Atmospheric and Oceanic Science Letters (2024). DOI: 10.1016/j.aosl.2024.100512
Provided by
Chinese Academy of Sciences
Citation:
Constructing a cross-component background error covariance for strongly coupled land-atmosphere data assimilation (2024, June 28)
retrieved 28 June 2024
from https://phys.org/news/2024-06-component-background-error-covariance-strongly.html
This document is subject to copyright. Apart from any fair dealing for the purpose of private study or research, no
part may be reproduced without the written permission. The content is provided for information purposes only.