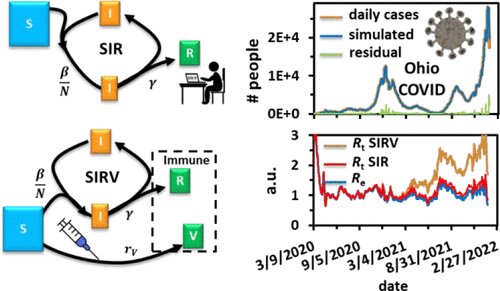
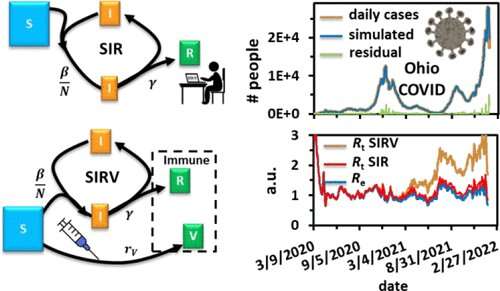
Chemist Jixin Chen looked at the rapid spread of COVID-19 early in the pandemic and saw a novel opportunity for his kinetics lab, where they study the rates of reactions.
The first time he ran the lab in spring 2021, undergraduate students concluded that social regulations such as lockdowns, face masks and social distancing were effective ways to slow the speed of the spread of COVID. But they also discovered the limitations of modeling, noting that a large number of confirmed cases didn’t necessarily associate with an increasing rate of spread.
Students in the next lab wrote in an ACS Omega journal article on their experience that researchers should continue this work when infection and vaccination rate became significant.
And that’s just what happened. The spring 2022 lab students extended the mathematical model to make predictions on the rate of spread of COVID-19 in the United States with massive vaccination.
They also ran the model for the state of Ohio through fall 2022—correctly predicting the upsurge in cases the state is experiencing at the end of summer.
The second group of lab students also wrote up their lab experience, this time seeing it published in the Journal of Chemical Education. All the students walked away from the lab with the requisites filled. But they also could add several lines to their resumes—for modeling software experience, data analysis skills, and a journal publication.
In spring 2021 when the world went remote, using the COVID model enabled Chen’s students to work on their own computers with publicly accessible data and software.
It worked so well that undergraduate students submitted a journal article on their experience, noting, “The viral spreading model is complicated but parameters, such as its reproduction number, Rt, can be estimated with the susceptible, infectious, or recovered model. COVID-19 data for many states and countries are widely available online. This provides an opportunity for the students to analyze its spreading kinetics remotely.”
Chen noted that COVID modeling provided an advantage when it came to explaining the steady-state approximation for some models in the textbook. The students noted that they benefitted by exploring the simulation function of commonly used software Excel.
“The most surprising and fun thing for me was how research can be accessible. We only used resources and data from free websites, but from there we were able to push further and dive into something so relevant to today’s society,” said Emma Lintelman, a rising senior chemistry major with a biological sciences minor in the College of Arts & Sciences.
In spring 2022, Chen and his students took the numerical simulation of kinetics and regression modeling even further.
“The first time we used this approach, the students were able to apply the kinetic techniques learned in physical chemistry to analyze an ongoing real-world problem through a remote learning environment,” Chen said. “This year, another group of undergraduate students led by graduate students Dylan Smith and Tharushi Ambagaspitiya did the same practice and extended the mathematical model to predict COVID-19 spread in the U.S. with massive vaccination.”
In the lab, the susceptible-infectious-recovery (SIR) model and the SIR-vaccinated (SIRV) model are explained to the students and are used to analyze the COVID-19 spread data from the U.S. Centers for Disease Control and Prevention (CDC). The basic reproduction number R0 and the real-time reproduction number Rt of COVID-19 are extracted by fitting the data with the models, which explains the spreading kinetics and provides a prediction of the spreading trend in a given state.
The students can quickly see the differences between the SIR model and the SIRV model, Chen said. The SIRV model considers the effect of vaccination, which helps explain the later stages of the ongoing pandemic.
The students also learned the predictive power of the models as they made predictions for the following months.
“I think the most surprising part of performing our COVID-19 kinetics simulation was seeing the drastic effects of varying the time-dependent reproduction number in our simulation,” said David McEwen, a senior majoring in chemistry and minoring in business. “This allowed us to directly simulate different levels of regulation of the virus through masking, social distancing, etc. By changing the number by a large amount, we were able to directly see with our data the increased or decreased spread rate of the virus, which was astounding at times.
“I think the most challenging parts for me were initially setting up our simulation parameters and fitting the simulated data to the collected case data. Fitting the simulated data to the actual case numbers sometimes required precise adjustment and took some time,” McEwen said.
Lintelman concurred.
“The most challenging part for me was working out the bugs in our formulas,” she said. “This can be tricky when you’ve been staring at your data for hours. It all just starts to swirl around in the mind, but that’s just when you need to come back to it later when you have a clear mind.”
Incidence of COVID-19 was eight times higher in unvaccinated vs. vaccinated students
Kelle D. Hart et al, Remote Learning of COVID-19 Kinetic Analysis in a Physical Chemistry Laboratory Class, ACS Omega (2021). DOI: 10.1021/acsomega.1c04842
Dylan K. Smith et al, Teaching Undergraduate Physical Chemistry Lab with Kinetic Analysis of COVID-19 in the United States, Journal of Chemical Education (2022). DOI: 10.1021/acs.jchemed.2c00416
Ohio University
Citation:
Chemistry lab students predict spread of COVID-19 with kinetics models (2022, August 11)
retrieved 11 August 2022
from https://phys.org/news/2022-08-chemistry-lab-students-covid-kinetics.html
This document is subject to copyright. Apart from any fair dealing for the purpose of private study or research, no
part may be reproduced without the written permission. The content is provided for information purposes only.