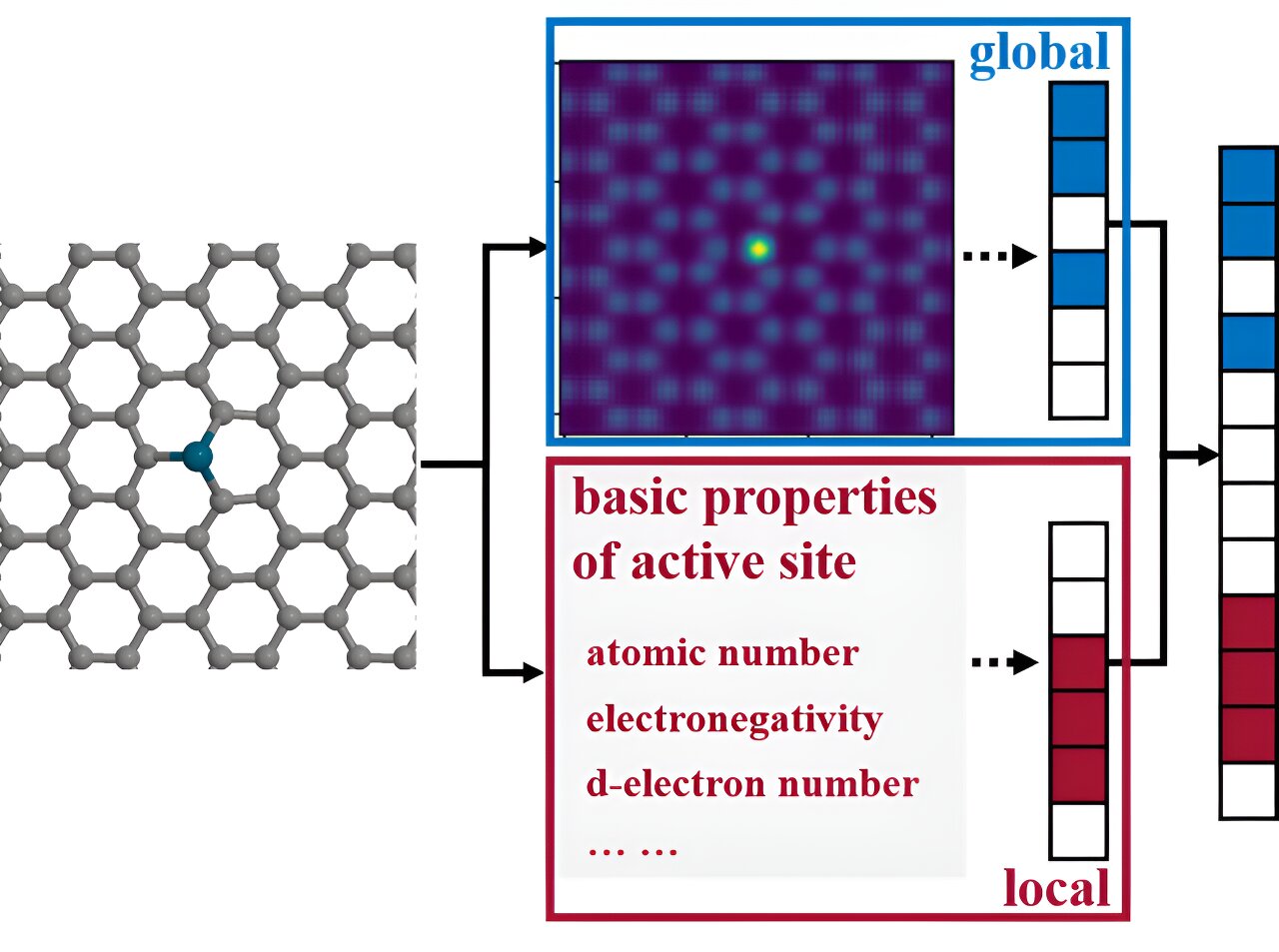
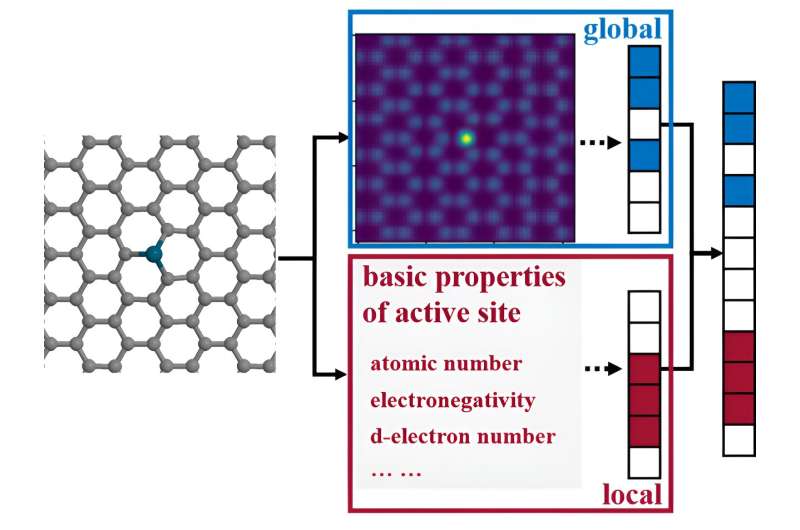
The fine structure of the catalytic surface has a significant impact on structural sensitive reactions, and high-throughput (HT) screening and machine learning (ML) are believed to effectively explore the potential rules of these effects and accelerate the developing of the catalyst. However, reported ML frameworks are too coarse to make a precise prediction of the catalytic performance.
Currently, the two commonly used conversion methods are descriptors and graphs. However, the construction of descriptors often ignores atomic connections, making it difficult for ML models to capture detailed geometrical information most relevant to catalytic performance.
The graph-based ML model inevitably loses the geometric arrangement information of adsorption sites during the process of updating nodes, and the complexity of the message passing neural network leads to its insensitivity to electronic or geometric structures and poor interpretability. Therefore, there is still a lack of interpretable ML frameworks that can simultaneously capture the features of electronic and geometric fine structures in heterogeneous catalysis.
Recently, a research team led by Prof. Yong Wang from Zhejiang University, China, created a data augmented convolutional neural network (CNN) ML framework called GLCNN, which combines “global + local” features. This framework can capture the original fine structures without complicated encoding methods by transforming catalytic surfaces and adsorption sites into two-dimensional grids and one-dimensional descriptors, respectively.
The addition of data augmentation (DA) can expand the dataset and alleviate overfitting caused by insufficiency of chemical datasets. The GLCNN framework accurately predicted and distinguished the adsorption energies of OH on a set of analogous carbon-based transition metal single-atom catalysts (TMSACs) with a mean absolute error (MAE) of less than 0.1 eV, ranking the best result of popular models trained on large datasets so far. The results were published in Chinese Journal of Catalysis .
Comparing GLCNN with descriptor or graph-based models, it was found that the comparison model cannot accurately predict the OH adsorption energy of catalysts containing IB and IIB transition metals or cis/trans configurations. The prediction performance of the GLCNN model is significantly better than that of the comparison model, indicating that the combination of grids and descriptors can better reflect the electronic and fine geometrical information of catalytic active centers.
Unlike conventional CNN and descriptor-based ones with one-sided feature extraction, this fine-structure sensitive ML framework can extract the key factors that affect catalytic performance from both geometric and chemical/electronic features, such as symmetry and coordination elements, through unbiased interpretable analysis.
The analysis of feature importance for descriptors part indicates that the electronic structure and symmetry element of adsorption sites are crucial, and the importance of metals is stronger than their coordination environment. Visualization analysis on each layer indicates that GLCNN can automatically extract geometrical information of chemical structures that conform to human intuition.
As the layers deepen, GLCNN gradually seeks the direction of feature extraction based on basic catalytic knowledge, extracting more abstract high-dimensional features that are conducive to adsorption energy prediction. This framework provides a feasible solution for high-precision HT screening of heterogeneous catalyst with a broad physical and chemical space.
More information:
Yuzhuo Chen et al, Fine-structure sensitive deep learning framework for predicting catalytic properties with high precision, Chinese Journal of Catalysis (2023). DOI: 10.1016/S1872-2067(23)64467-5
Provided by
Chinese Academy of Sciences
Citation:
Fine-structure sensitive deep learning framework for prediction of catalytic properties with high precision (2023, September 6)
retrieved 6 September 2023
from https://phys.org/news/2023-09-fine-structure-sensitive-deep-framework-catalytic.html
This document is subject to copyright. Apart from any fair dealing for the purpose of private study or research, no
part may be reproduced without the written permission. The content is provided for information purposes only.