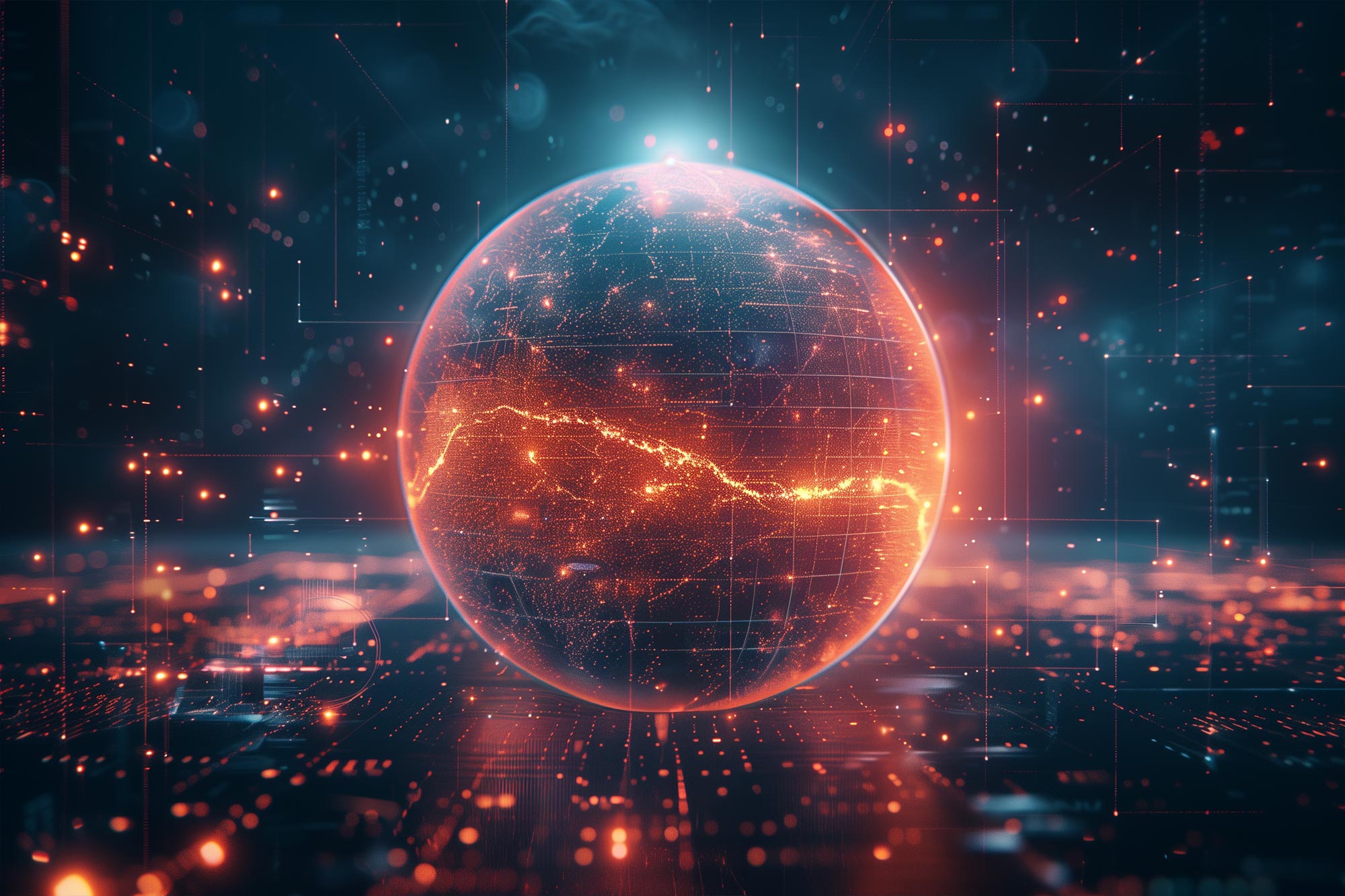
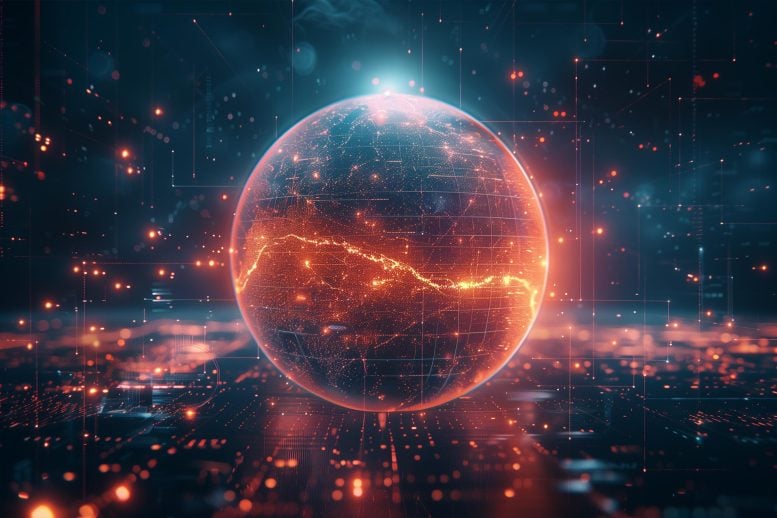
Researchers have achieved a significant advancement in understanding exoplanet atmospheres through the use of physics-informed neural networks (PINNs), which allow for more precise modeling of light scattering effects, particularly from clouds.
This breakthrough, stemming from collaborative efforts among several prestigious institutions, leverages AI to improve the analysis of spectral data from exoplanets, particularly benefiting from new data from the James Webb Space Telescope.
Breakthrough in Exoplanet Atmosphere Analysis
Scientists from LMU, the ORIGINS Excellence Cluster, the Max Planck Institute for Extraterrestrial Physics (MPE), and the ORIGINS Data Science Lab (ODSL) have achieved a significant breakthrough in analyzing exoplanet atmospheres. By employing physics-informed neural networks (PINNs), they have enhanced the modeling of complex light scattering within these atmospheres, achieving greater precision than ever before. This innovative approach offers new insights into the role of clouds and could dramatically enhance our knowledge of distant worlds.
When distant exoplanets pass in front of their star, they block a small portion of the starlight, while an even smaller portion penetrates the planetary atmosphere. This interaction leads to variations in the light spectrum, which mirror the properties of the atmosphere such as chemical composition, temperature, and cloud cover.
To be able to analyze these measured spectra, however, scientists require models that are capable of calculating millions of synthetic spectra in a short time. Only by subsequently comparing the calculated spectra with the measured ones do we obtain information about the atmospheric composition of the observed exoplanets. And what is more, the highly detailed new observations coming from the James Webb Space Telescope (JWST) necessitate equally detailed and complex atmospheric models.
Enhanced Modeling With Physics-Informed Neural Networks
A key aspect of exoplanet research is the light scattering in the atmosphere, particularly the scattering off clouds. Previous models were unable to satisfactorily capture this scattering, which led to inaccuracies in the spectral analysis. Physics-informed neural networks offer a decisive advantage here, as they are capable of efficiently solving complex equations. In the just-published study, the researchers trained two such networks. The first model, which was developed without taking light scattering into account, demonstrated impressive accuracy with relative errors of mostly under one percent. Meanwhile, the second model incorporated approximations of so-called Rayleigh scattering – the same effect that makes the sky seem blue on Earth. Although these approximations require further improvement, the neural network was able to solve the complex equation, which represents an important advance.
Advantages of Interdisciplinary Collaboration
These new findings were possible thanks to a unique interdisciplinary collaboration between physicists from LMU Munich, the ORIGINS Excellence Cluster, the Max Planck Institute for Extraterrestrial Physics (MPE), and the ORIGINS Data Science Lab (ODSL), which specializes in the development of new AI-based methods in physics.
“This synergy not only advances exoplanet research, but also opens up new horizons for the development of AI-based methods in physics,” explains lead author of the study David Dahlbüdding from LMU. “We want to further expand our interdisciplinary collaboration in the future to simulate the scattering of light off clouds with greater precision and thus make full use of the potential of neural networks.”
Reference: “Approximating Rayleigh scattering in exoplanetary atmospheres using physics-informed neural networks” by David Dahlbüdding, Karan Molaverdikhani, Barbara Ercolano and Tommaso Grassi, 02 August 2024, Monthly Notices of the Royal Astronomical Society.
DOI: 10.1093/mnras/stae1872