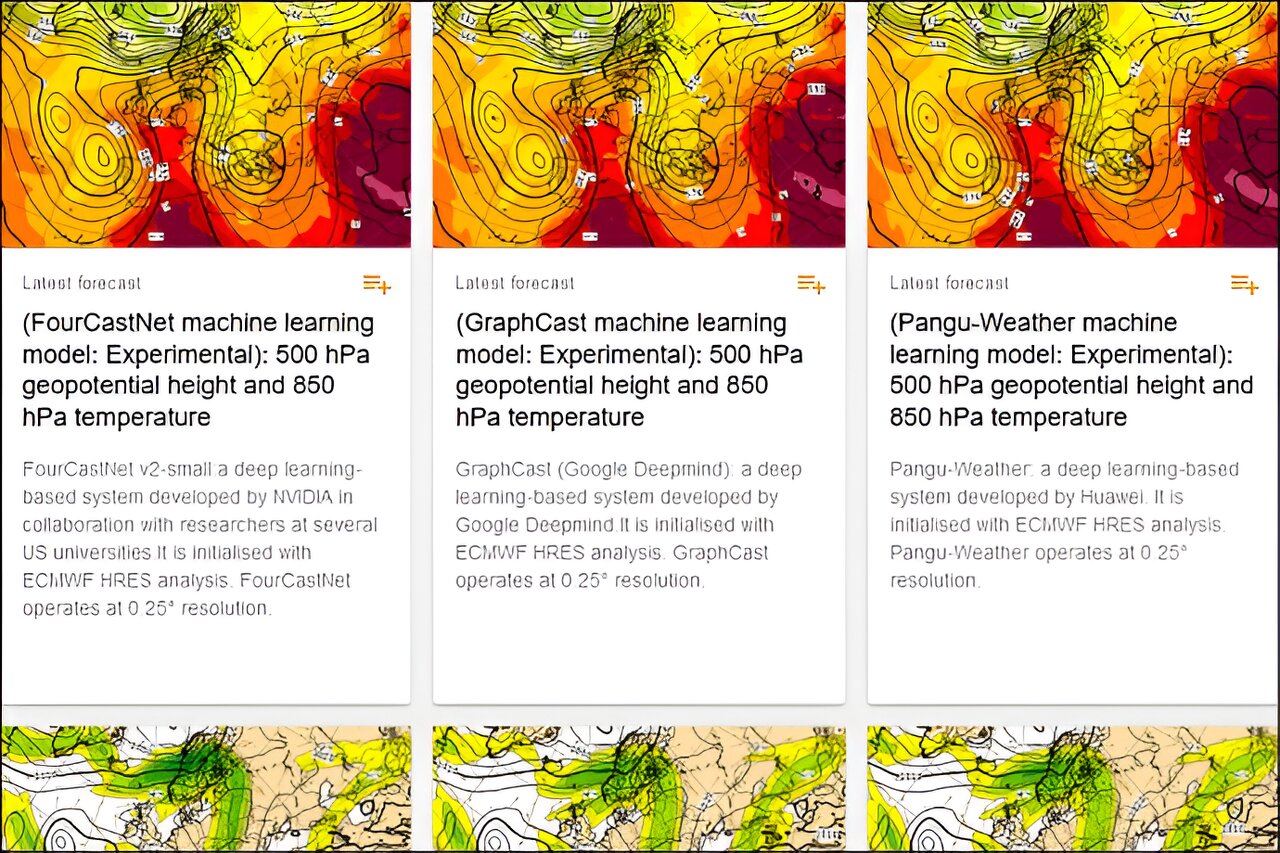
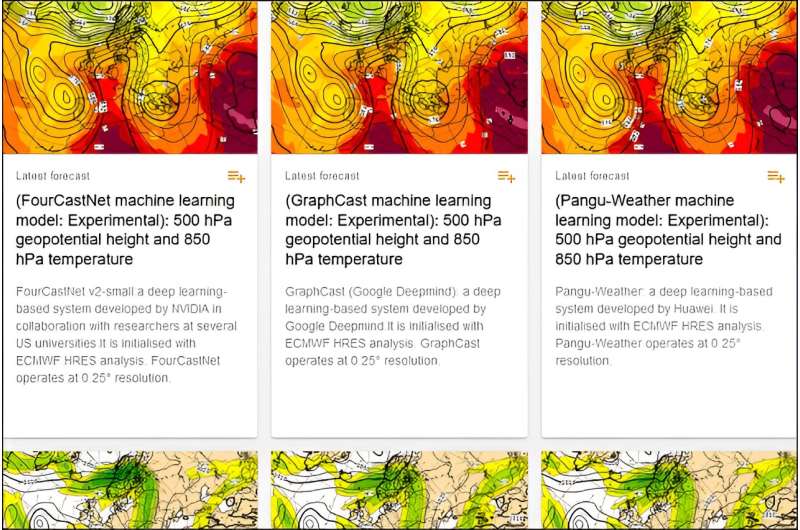
Developments in machine learning are continuing at breathtaking pace, both inside and outside of weather forecasting. To help assess machine learning weather forecasts from different sources, we now show a range of them in ECMWF’s charts catalog.
We are also exploring the use of machine learning models to produce ensemble forecasts, which present the range of future weather possibilities.
European Centre for Medium-Range Weather Forecasts (ECMWF) has been highlighting the rapid rise of data-driven forecasts. These are produced by machine-learning-based forecasting models, created by innovators such as NVIDIA, Huawei and Google DeepMind.
The ERA5 reanalysis, produced by the Copernicus Climate Change Service (C3S) implemented by ECMWF, is the dataset used to train these models. They can make accurate 10-day forecasts when provided with good initial conditions.
This summer, we focused on analyzing Huawei’s Pangu-Weather to understand its strengths and weaknesses, with results collated in a submitted manuscript. At this early stage in the technology, some results are already displaying comparable skill to ECMWF’s Integrated Forecasting System (IFS), which is an exciting achievement.
With the recent array of promising and fascinating results, which could be a game changer for our science, it is important to take time to properly assess the operational value of these models.
“FourCastNet was the first AI-based weather forecasting system at 0.25 degrees and the first to be open-sourced. Our new version has significantly improved medium-range skill and long-term stability, since the model respects spherical geometry. As we scale up further, we are excited to continue using the framework of neural operators that enable super-resolution or downscaling natively,” said Anima Anandkumar from NVIDIA’s Earth-2 team.
Feedback sought
We believe the optimal strategy is to present these machine learning systems alongside tried and tested operational forecasts to a wide range of users with real applications, and to invite feedback on the systems’ operational readiness and performance. We consider factors such as forecast accuracy, reliability, uncertainty, and communication as essential for assessing the quality and effectiveness of meteorological products.
To this end, daily forecasts starting from ECMWF initial conditions and using NVIDIA’s FourCastNet, Huawei’s Pangu-Weather and Google DeepMind’s GraphCast are now freely available to view on ECMWF’s public charts pages. Details on each of these models can be found on the ECMWF website.
We show a range of forecast products, depending on which variables these models produce—for example, not all of them predict precipitation. We provide additional guidance about the quality of these forecasts by adding charts to our verification section to show the skill levels of these models for different users and applications in a comparable environment. We encourage everyone to get in touch to share their most interesting findings from these models.
“Open science is the key to innovation, collaboration, and discovery. By sharing data, methods, and results, and making them easily accessible and comparable, we can accelerate the progress of science that can ultimately benefit society,” says ECMWF Director of Forecasts Florian Pappenberger.
We developed a software solution that lets the public access these models with a single command to enhance transparency and reproducibility. This software package can automatically retrieve the initial conditions for a forecast from different places, including our MARS archive, the Climate Data Store as well as local file storage.
Through a plug-in system, we make the different models installable and accessible to anyone. This is freely released as ai-models, with public plugins for FourCastNet, Pangu-Weather and GraphCast.
“We are delighted to know that Pangu-Weather appears on ECMWF’s charts. This means that everyone in the world can access Pangu’s results without downloading any data and running any code,” said Lingxi Xie, one of the authors of Pangu-Weather.
Future plans
From our analysis, we see evidence that machine-learning-based forecasts will have a role to play in future operational forecasting systems. However, there are still interesting challenges ahead. For example, these models do not yet produce ensemble forecasts, something we believe is key for delivering valuable forecasts for medium-range timescales.
To address these challenges, earlier this summer ECMWF embarked on a project to create a probabilistic machine learning forecasting system, AIFS. This will build on all the research carried out over the last few years in the field of machine learning, but also on our decades-long expertise in building and verifying ensemble systems.
“Weather forecasting systems have a profound and beneficial impact on humanity as a whole. GraphCast, Google DeepMind’s machine-learning global weather model, is our attempt at advancing this critical technology. GraphCast is available on github, and sharing its live forecasts is an important next step in building trust in its performance, supporting the weather and machine learning communities, and getting feedback to further improve the next generation of models,” according to the Google DeepMind GraphCast team.
An overview paper is published on the arXiv preprint server.
More information:
Zied Ben-Bouallegue et al, The rise of data-driven weather forecasting, arXiv (2023). DOI: 10.48550/arxiv.2307.10128
Journal information:
arXiv
Provided by
European Centre for Medium-Range Weather Forecasts (ECMWF)
Citation:
How AI models are transforming weather forecasting: A showcase of data-driven systems (2023, September 6)
retrieved 7 September 2023
from https://phys.org/news/2023-09-ai-weather-showcase-data-driven.html
This document is subject to copyright. Apart from any fair dealing for the purpose of private study or research, no
part may be reproduced without the written permission. The content is provided for information purposes only.