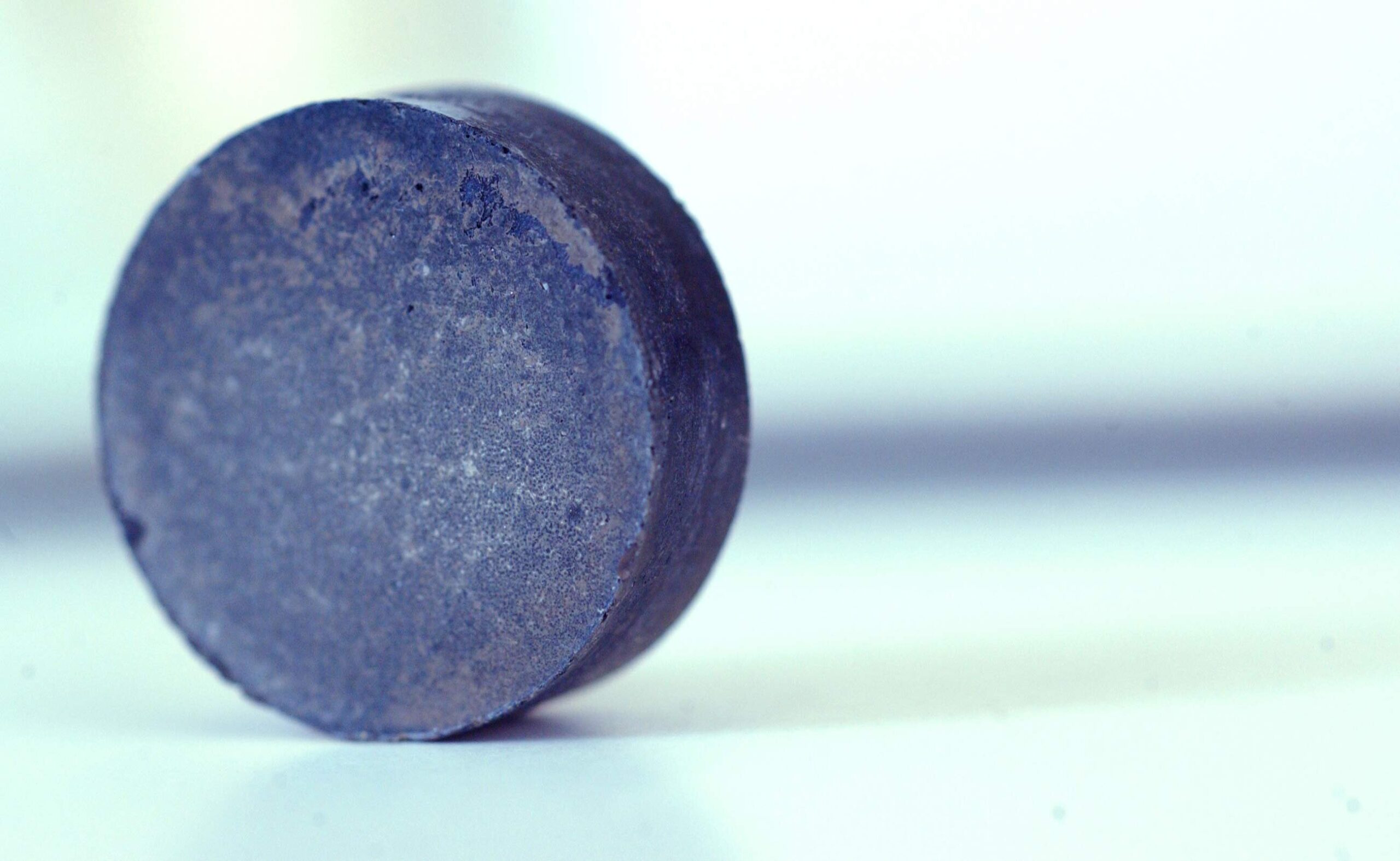
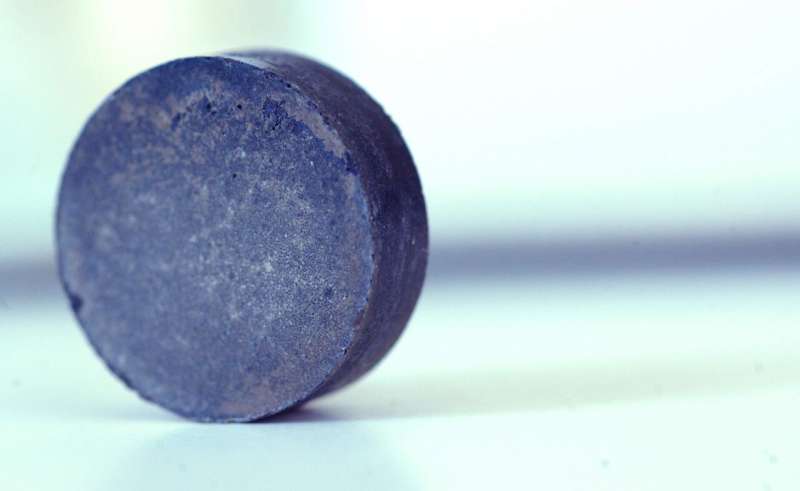
A team of scientists from Ames National Laboratory has developed a new machine learning model for discovering critical-element-free permanent magnet materials. The model predicts the Curie temperature of new material combinations. It is an important first step in using artificial intelligence to predict new permanent magnet materials. This model adds to the team’s recently developed capability for discovering thermodynamically stable rare earth materials. The work is published in Chemistry of Materials.
High performance magnets are essential for technologies such as wind energy, data storage, electric vehicles, and magnetic refrigeration. These magnets contain critical materials such as cobalt and rare earth elements like neodymium and dysprosium. These materials are in high demand but have limited availability. This situation is motivating researchers to find ways to design new magnetic materials with reduced critical materials.
Machine learning (ML) is a form of artificial intelligence. It is driven by computer algorithms that use data and trial-and-error algorithms to continually improve its predictions. The team used experimental data on Curie temperatures and theoretical modeling to train the ML algorithm. Curie temperature is the maximum temperature at which a material maintains its magnetism.
“Finding compounds with the high Curie temperature is an important first step in the discovery of materials that can sustain magnetic properties at elevated temperatures,” said Yaroslav Mudryk, a scientist at Ames Lab and senior leader of the research team. “This aspect is critical for the design of not only permanent magnets but other functional magnetic materials.”
According to Mudryk, discovering new materials is a challenging activity because the search is traditionally based on experimentation, which is expensive and time-consuming. However, using a ML method can save time and resources.
Prashant Singh, a scientist at Ames Lab and member of the research team, explained that a major part of this effort was to develop an ML model using fundamental science. The team trained their ML model using experimentally known magnetic materials. The information about these materials establishes a relationship between several electronic and atomic structure features and Curie temperature. These patterns give the computer a basis for finding potential candidate materials.
To test the model, the team used compounds based on cerium, zirconium, and iron. This idea was proposed by Andriy Palasyuk, a scientist at Ames Lab and member of the research team. He wanted to focus on unknown magnet materials based on earth-abundant elements. “The next super magnet must not only be superb in performance, but also rely on abundant domestic components,” said Palasyuk.
Palasyuk worked with Tyler Del Rose, another scientist at Ames Lab and member of the research team, to synthesize and characterize the alloys. They found that the ML model was successful in predicting the Curie temperature of material candidates. This success is an important first step in creating a high-throughput way of designing new permanent magnets for future technological applications.
“We are writing physics-informed machine learning for a sustainable future,” said Singh.
More information:
Prashant Singh et al, Physics-Informed Machine-Learning Prediction of Curie Temperatures and Its Promise for Guiding the Discovery of Functional Magnetic Materials, Chemistry of Materials (2023). DOI: 10.1021/acs.chemmater.3c00892
Provided by
Ames National Laboratory
Citation:
Researchers use AI to find new magnetic materials without critical elements (2023, September 5)
retrieved 5 September 2023
from https://phys.org/news/2023-09-ai-magnetic-materials-critical-elements.html
This document is subject to copyright. Apart from any fair dealing for the purpose of private study or research, no
part may be reproduced without the written permission. The content is provided for information purposes only.